Minimizing Risk With Big Data & Analytics
Anti-Money Laundering (AML) is a global risk for governments and financial institutions. Many financial institutions such as banks and government departments like the United States Department of State deal with AML. AML is also a topic covered in academic research.
The U.S. Department of State defines a major Money Laundering country as a country “whose financial institutions engage in currency transactions involving significant amounts of proceeds from international narcotics trafficking.” Given that fact AML occurs in many countries across the globe from Argentina to Canada to Mexico and more.
However, the risk level in Anti-Money Laundering differs by country. The Basel Institute on Governance published its third Basel Anti-Money Laundering Index that ranks countries based on their AML and Terrorist Financing risk. The chart below shows the Top 15 riskiest AML countries according to Basel AML Index:
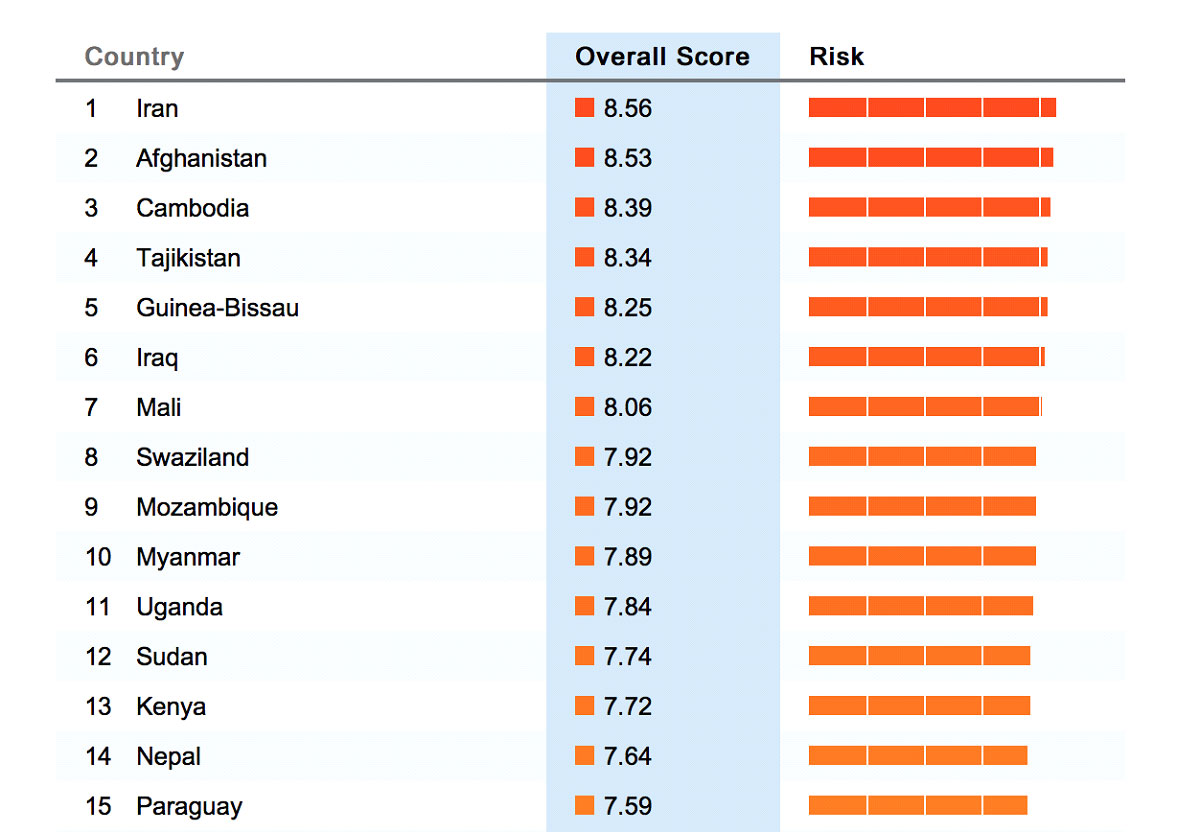
Financial institutions such as banks need to minimize risk where Anti-Money Laundering is one of the biggest risks. In order for companies to analyze and minimize AML it is important for the analyst to:
- Understand the Customer
- Understand the Nature of the Transaction
- Location of Transaction
- Be Aware of Cultural Differences
- Acknowledge Economic Sanctions (Cuba, North Korea and etc.)
Analyzing AML with Hadoop
Since the recent popularity of Big Data and demand for analytics, analyzing risk in AML has become much more economical and efficient. Analyzing Anti-Money Laundering across the globe means larger amounts of data for a business to store and examine. Financial institutions such as American Express, JP Morgan and Barclays have started to solve this issue of data storage by using Apache Hadoop, an open source software framework that allows for distributed storage and processing of large data sets.
Prior to Hadoop companies used traditional databases that did not support very large volumes of data and analysis was solely based off of small data sets. The introduction of Hadoop allows access to large volumes of data and enables the company to extract and transform that data into information used for decision-making, fraud and AML detection.
Due to this advantage Hadoop can store historical data. This information is especially helpful for financial institutions analyzing risk. With historical data a bank can create prediction models to control risk and can also run various algorithms, such as matching, to detect patterns in fraud behavior and provide a model to decrease the levels of risky behavior.
Bluestar Technology Solutions provides analysts, data scientists and developers the technological and statistical skills needed to conduct this analysis and provides knowledge of finance and risk management needed to create prediction models and use cases. Having this knowledge and technological skills enables the firm to successfully minimize the risk of Anti-Money Laundering.